Seminar Series. Adam Sadilek: Machine Learned Epidemiology
Work in computational epidemiology to date has been limited by coarseness and lack of timeliness of observational data. Most existing models are based on hand-curated statistics that are often delayed, expensive to collect, and cover only limited jurisdictions. Our goal is to lift the state of the art in epidemiology to a new qualitative state, where real-time health predictions become feasible and actionable. We do this at scale by applying (federated) machine learning and secure aggregation to online data to infer what likely contributed to the contagion. In this talk, I will sample current projects at Google focusing on privacy-first epidemiology research and recent publications.
Preprint Privacy-first health research with federated learning
Nature Feb 2021: Lymelight: forecasting Lyme disease risk using web search data
Nature May 2020: Mapping global variation in human mobility
Science Sept 2020: Assessing the impact of coordinated COVID-19 exit strategies across Europe
Nature Oct 2019: Hierarchical organization of urban mobility and its connection with city livability
Nature Nov 2018: Machine-learned epidemiology: real-time detection of foodborne illness at scale
Adam Sadilek focuses on large-scale machine learning applied to health and ecology at Google Research. Before that, he worked on speech understanding at Google[x]. Prior to joining Google, Adam was a co-founder of Fount.in, a machine learning startup providing automated text understanding.
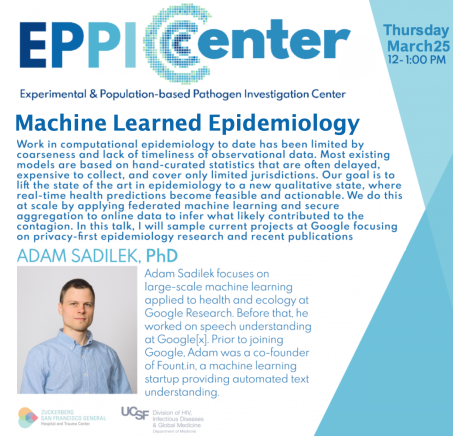